1. What Are Multi-Agent AI Systems?
A Multi-Agent AI System consists of multiple autonomous AI agents that work together within a shared environment to achieve common or individual goals. Instead of having one AI handling everything, multiple specialized AI models interact and collaborate to improve efficiency.
Think of them as AI coworkers because some handle customer queries, others optimize logistics, and some even monitor security threats. Unlike human coworkers, they don’t take coffee breaks or forget deadlines. 😉
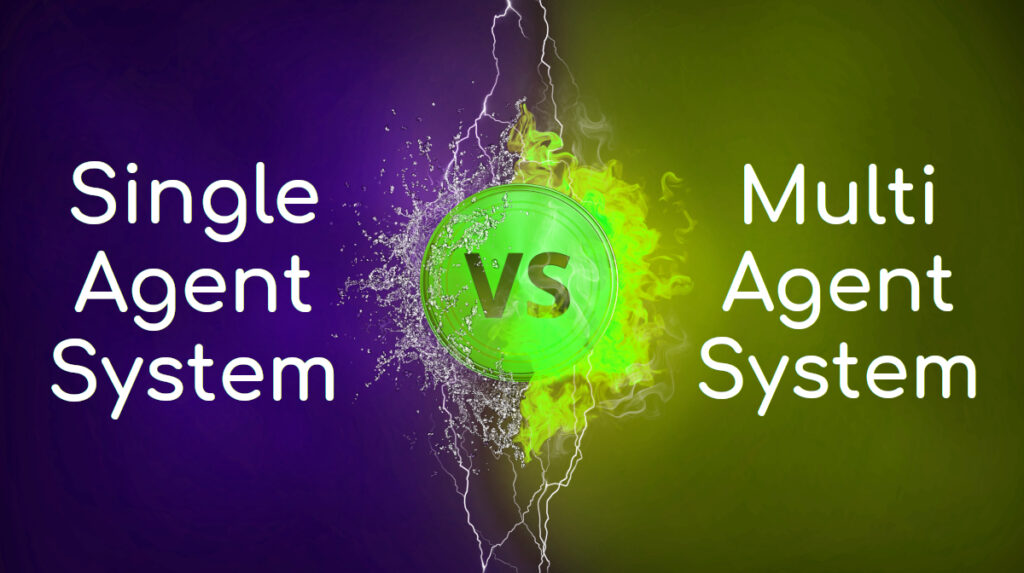
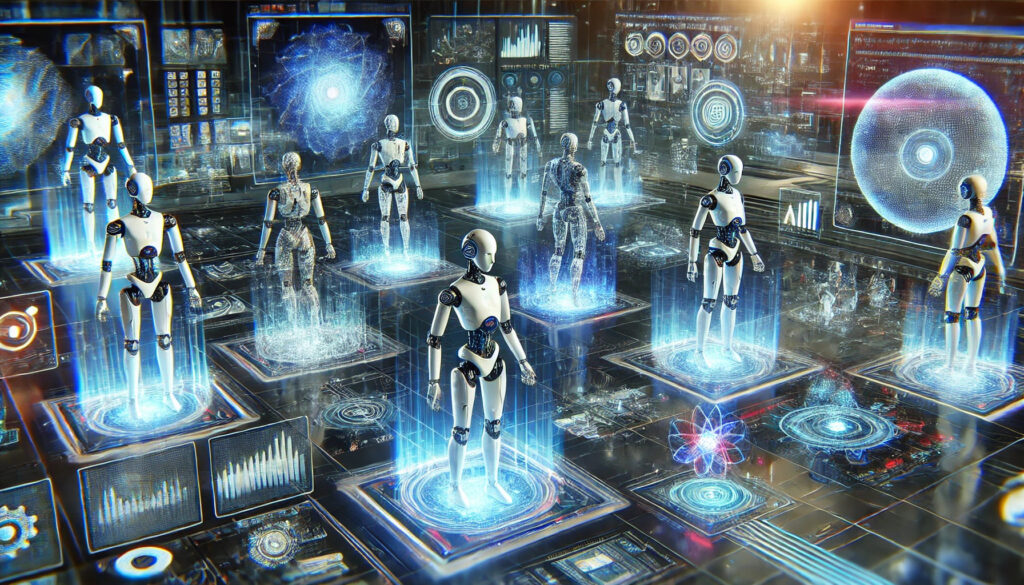
How Do They Work?
- Autonomous Decision-Making: Each agent operates independently but shares insights with others.
- Task Distribution: AI agents divide tasks based on expertise, just like employees in different departments.
- Continuous Learning: The system adapts and improves over time, making it smarter with experience.
2. How Enterprises Are Using Multi-Agent AI Systems
1. Customer Support Automation
Gone are the days of slow customer service responses. Companies now use multiple AI agents to handle queries, process transactions, and offer personalized recommendations. While one AI agent responds to questions, another analyzes sentiment and urgency to escalate issues automatically.
- Example: E-commerce giants use Multi-Agent AI to offer real-time chat support, product suggestions, and order tracking without human intervention.
2. Supply Chain Optimization
Supply chains are complex, and one wrong move can cause inventory shortages or delivery delays. Multi-Agent AI systems monitor logistics, demand forecasting, and real-time transportation tracking to avoid such disruptions.
- Example: AI agents collaborate to manage warehouse inventory, predict demand spikes, and optimize delivery routes—ensuring your online order actually arrives on time (unlike that one package that’s still “out for delivery” from last year). 😉
3. Financial Decision-Making & Fraud Detection
Financial institutions deploy AI agents to analyze stock markets, detect fraud, and automate transactions. These agents evaluate risks, anomalies, and suspicious activity, alerting security teams in real-time.
- Example: AI in banking detects unusual transactions before fraud occurs, reducing financial losses and protecting customers. No more random charges from “Suspicious Store 101.” 😉
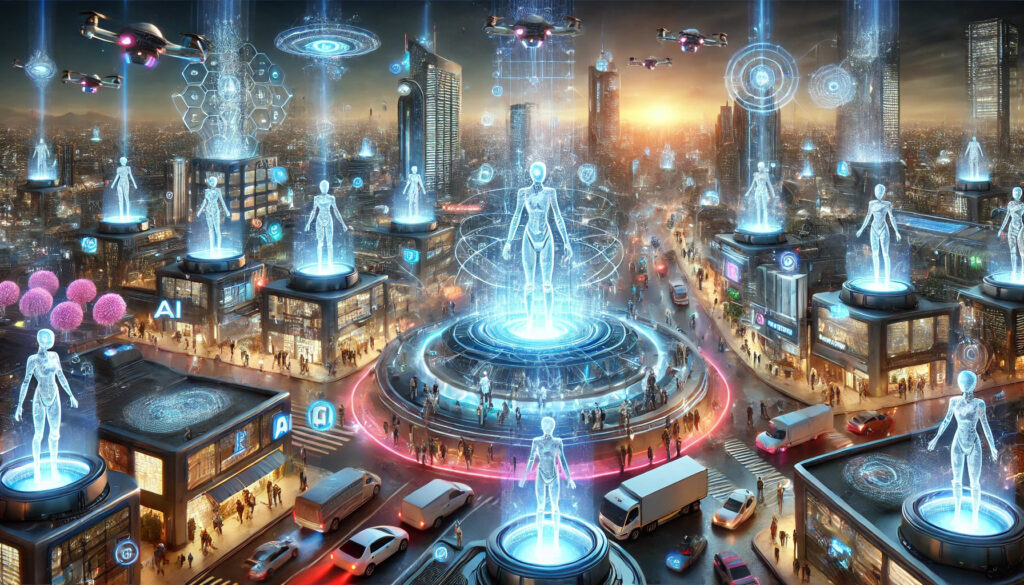
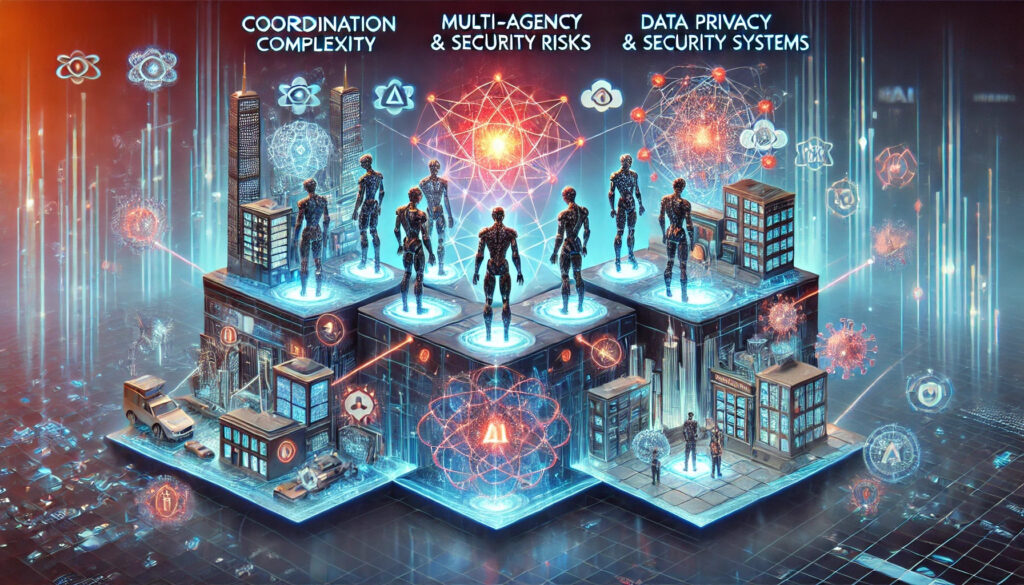
3. Challenges of Multi-Agent AI Systems
1. Coordination Complexity When multiple AI agents collaborate, miscommunication can still happen (just like in human teams). Ensuring seamless decision-making and data flow requires careful system design.
- Solution: Businesses implement reinforcement learning to improve AI coordination over time.
2. Data Privacy & Security Risks
More AI agents mean more data-sharing, increasing the risk of breaches. Security must be airtight to prevent sensitive enterprise data from leaking.
- Solution: Companies encrypt AI communications and use secure access controls to protect sensitive information.
3. Integration with Legacy Systems
Many enterprises rely on outdated IT infrastructure that doesn’t easily integrate with modern AI solutions. AI agents must be carefully integrated to avoid disrupting existing workflows.
- Solution: Organizations adopt hybrid AI models that gradually introduce AI automation while keeping legacy systems operational.
Conclusion
Multi-Agent AI Systems are transforming enterprises by automating complex processes, improving decision-making, and boosting operational efficiency. Whether it’s customer service, supply chain management, or fraud detection, AI agents are working together to optimize enterprise operations.
However, challenges like coordination, security, and integration need careful handling to maximize benefits. Businesses that successfully implement Multi-Agent AI Systems will have a significant competitive advantage in the future.
AI is no longer just about one assistant, it’s about an entire AI workforce working together!
Want to stay ahead of AI-driven automation? Subscribe to our newsletter for the latest AI insights, trends, and best practices. Let’s explore how AI can transform your business!