What Is Deep Learning?
Deep learning is the brainchild of neural networks, complex algorithms, and vast datasets. It mirrors human brain activity, enabling machines to process information with unprecedented depth. At its core are models like Convolution Neural Networks (CNNs), Recurrent Neural Networks (RNNs), and the ever-popular Generative Pre-trained Transformer (GPT).
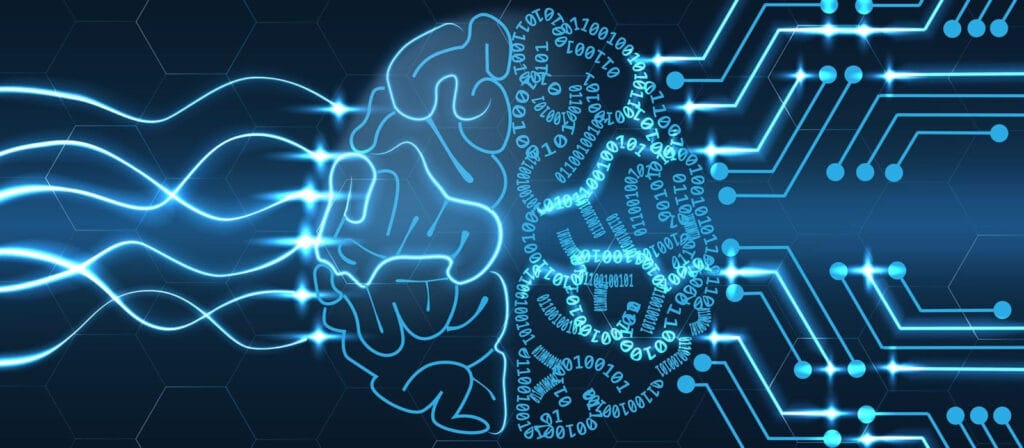
Deep Learning vs. Machine Learning
Distinguishing deep learning from its sibling, machine learning, is crucial. Deep learning, with its robust neural networks, tackles complex tasks, leaving standard machine learning models in its wake.
Deep Learning vs. Neural Networks
Neural networks form the backbone of deep learning, showcasing a brain-like architecture. Unlike traditional AI models, deep learning demands at least three neural networks, allowing for unparalleled sophistication.
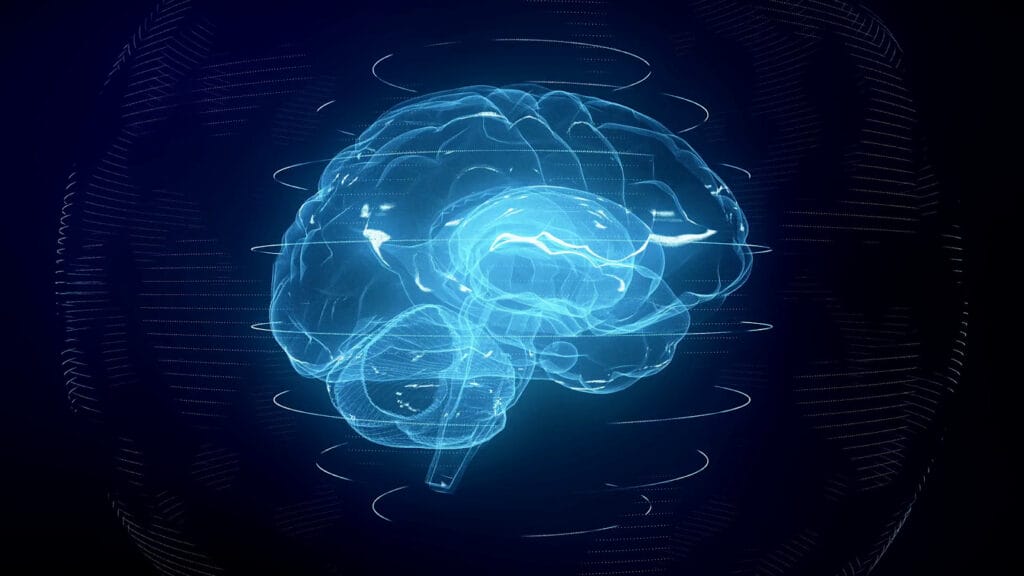
The Pros of Deep Learning
1. Capable of Different Learning Styles:
Deep learning models flaunt versatility, learning through various methods. From supervised and unsupervised learning to self-supervised and reinforcement learning, these models adapt, making them ideal for scalable automation.
2. Works Well With Unstructured Big Data:
Unstructured data poses a challenge for many AI models. Deep learning, however, effortlessly understands and applies large unstructured datasets to its training, eliminating the need for extensive data preparation.
3. Recognizes Complex Data Patterns:
The neural network design empowers deep learning models to grasp intricate data patterns and relationships. This human-like understanding fuels tasks like classification, summarization, and contextualized outputs.
4. High Levels of Scalability:
Mimicking the human brain's adaptability, deep learning models excel in scalability. Through strategies like transfer learning, they evolve to handle diverse business and personal tasks.
The Cons of Deep Learning
1. High Energy Consumption
The power-hungry nature of deep learning models demands substantial computing power, resulting in high energy consumption. This not only limits accessibility but also raises environmental concerns.
2. Expensive Infrastructure Components
Equipping deep learning models with GPUs and specialized chips comes at a cost. The scarcity of these components has led to a shortage, hindering the operation of advanced deep learning models.
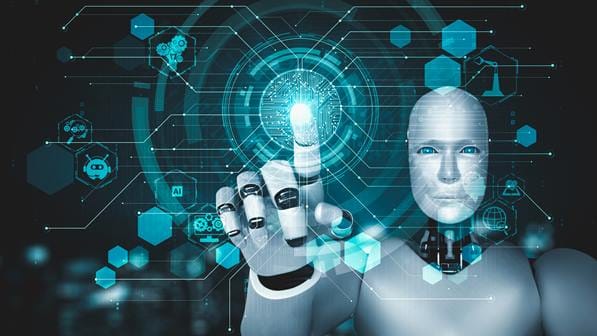
3. Limited Transparency
Understanding the outputs of deep learning models, especially those using unsupervised learning, remains a challenge. This lack of transparency hinders quality assurance and user understanding.
4. Security and Ethical Concerns
Deep learning introduces security and ethical worries. Limited visibility into training practices and data sources opens avenues for unauthorized access to sensitive data, raising cybersecurity issues.
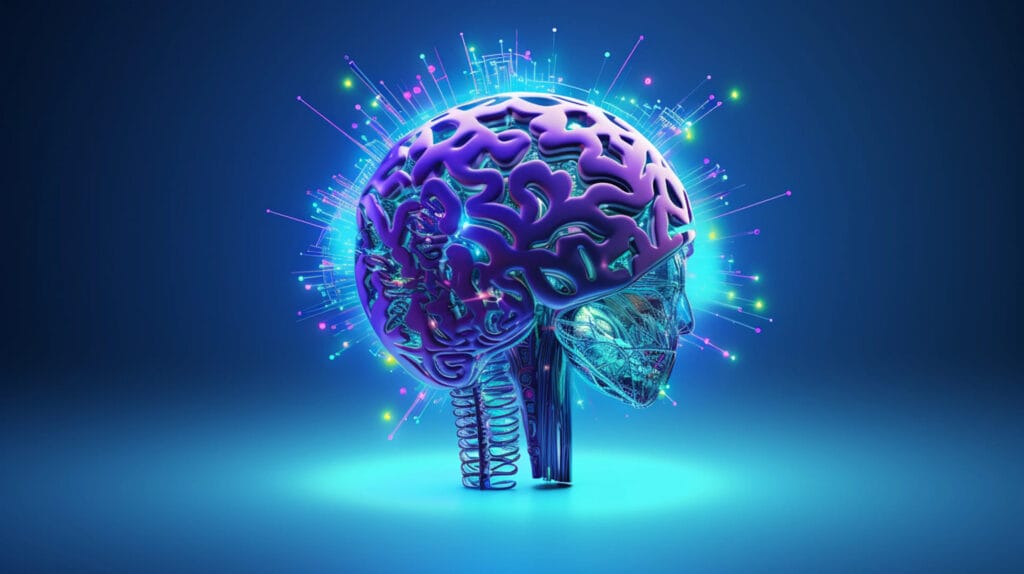
Bottom Line: Balancing Power and Responsibility
In conclusion, while deep learning is a formidable force in AI, it necessitates careful consideration. The advantages it brings to the table, from automation to understanding complex data, outweigh the challenges. To harness its potential responsibly, establishing policies and best practices is the key. Deep learning is not a problem to solve but a power to wield with care for a brighter technological future.