AI's Leap Forward: A Reality Check
The Moving Target Challenge
AI is a dynamic field, and staying at the forefront can be challenging. As McKinsey Global Institute research suggests, there's a noticeable divide between AI leaders and laggards. Leaders not only adopt AI more extensively but also plan to invest more in the near future.
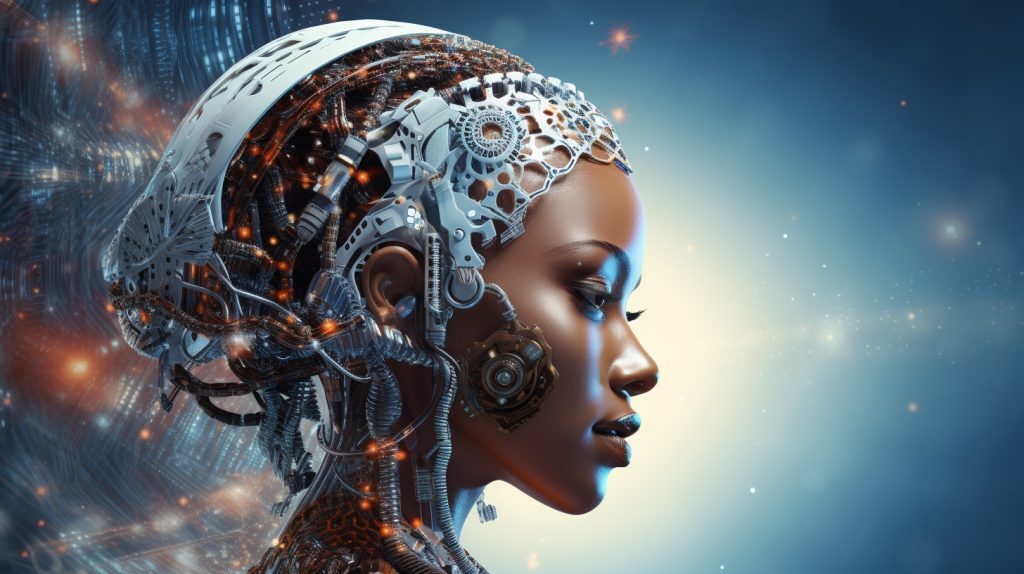
Technical and Organizational Hurdles
Success with AI requires a nuanced understanding. It's not just about where AI can enhance innovation and decision-making but also about recognizing its current limitations. These include technical challenges like data labeling and the need for massive training data sets, as well as organizational barriers like cultural resistance and a shortage of skilled personnel.
Overcoming Limitations: A Glimpse into the Future
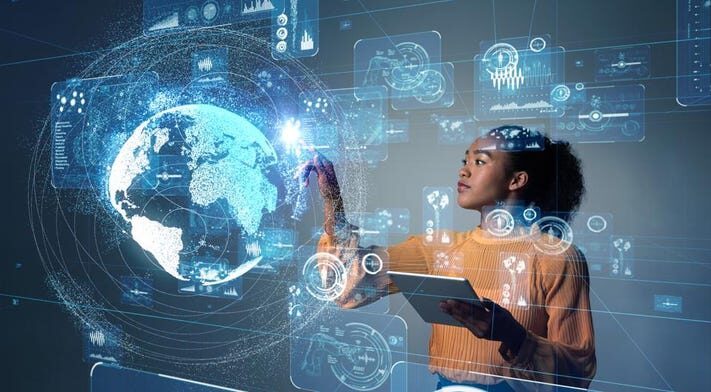
Limitation 1: Data Labeling Woes
The majority of AI models rely on supervised learning, demanding human-labeled data. This can be an arduous task, especially for complex applications like self-driving cars. Promisingly, techniques like reinforcement learning and generative adversarial networks (GANs) are emerging to reduce this dependency.
- Reinforcement Learning: Mimics a "carrot and stick" approach, where algorithms learn through trial and error.
- Generative Adversarial Networks (GANs): Involves two networks competing to refine their understanding of a concept, reducing the need for human-labeled data.
Limitation 2: Massive Training Data Sets
Deep learning craves extensive data sets, posing a challenge for many businesses. Enter one-shot learning, a technique allowing AI models to learn from a small number of real-world examples.
- One-shot Learning: Enables AI models to grasp a subject with minimal real-world demonstrations, potentially transforming various industries.
Limitation 3: The Explainability Conundrum
Understanding why an AI makes a specific decision is crucial, especially in sensitive areas. Novel approaches like local-interpretable-model-agnostic explanations (LIME) and attention techniques aim to enhance model transparency.
- Local-interpretable-model-agnostic explanations (LIME): Identifies crucial parts of input data for interpretable models.
- Attention Techniques: Visualize data segments considered most important during decision-making.
Limitation 4: Generalizability Struggles
AI models often struggle to apply learning from one circumstance to another. Transfer learning, the idea of applying knowledge from one task to a related one, offers a promising solution.
- Transfer Learning: Trains AI models for a specific task and then applies that learning to a similar activity.
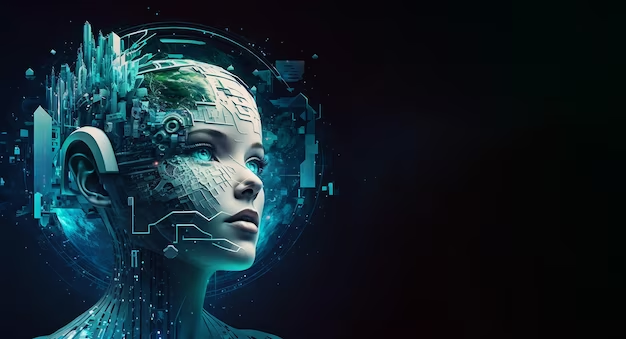
Limitation 5: Bias in Data and Algorithms
Biases in data and algorithms can lead to serious consequences. Recognizing and addressing biases is a pressing issue that requires a deep understanding of data-science techniques.
Navigating the AI Frontier: Tips for Leaders
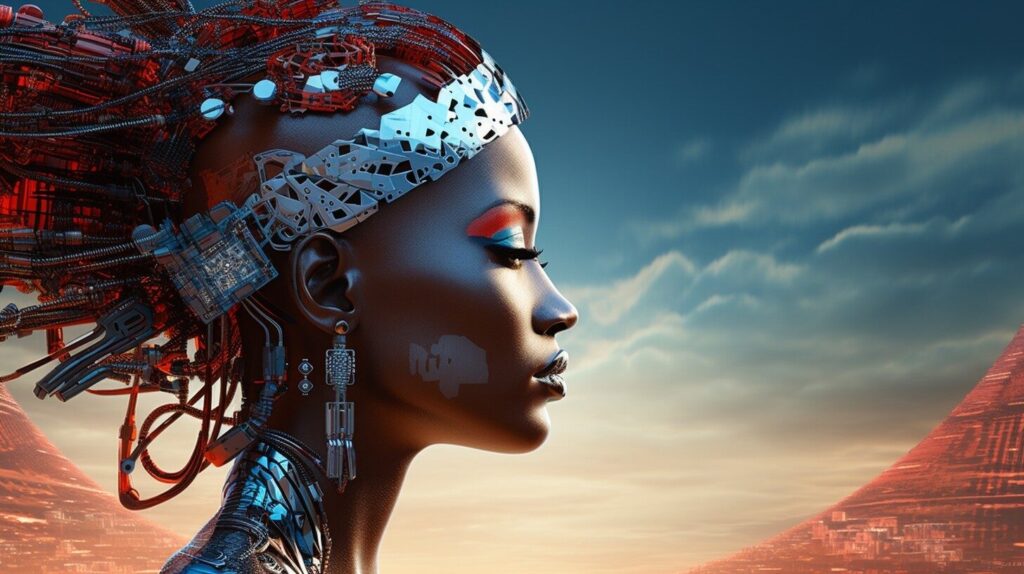
1. Stay Informed: Even if you're not knee-deep in technical details, a general understanding of AI capabilities and trends is crucial. Leverage your data science team, connect with AI pioneers, and attend conferences for firsthand insights.
2. Craft a Data Strategy: AI's power lies in data, so develop a comprehensive data strategy. Understand your data points, focus on data availability, and explore new techniques that promise to reduce data requirements.
3. Think Laterally: Explore ways to leverage AI solutions across different areas of your business. Encourage collaboration between business units to discover innovative uses for your AI solutions.
4. Be a Trailblazer: Don't just keep up with current AI technologies; aim to solve high-impact problems with cutting-edge techniques. Stay informed about emerging tools and models to gain a competitive advantage.
Conclusion
In conclusion, the promise of AI is vast, but so are the challenges. Leaders who understand the current landscape, embrace emerging solutions, and navigate the evolving AI frontier will be well-positioned to unlock its immense potential. It's not just about keeping up; it's about leading the way into the future of artificial intelligence.