1. Problem Spotting: Seeing the Real Issue
Imagine you're working with a mid-range hotel chain, and they're grappling with low ratings on their check-in process. Guests are unhappy, and it's affecting their return rate. At first glance, it seems like a check-in problem. But data scientists have a unique perspective. They can dig deeper and spot the real issues.
In this case, it took a bit of digging into customer surveys and some clever text analytics to reveal that the problem wasn't with check-in itself but with the overall hotel experience. Guests were frustrated with Wi-Fi, room keys, and cleanliness. The takeaway here? Solving what's in front of you might mean missing more significant opportunities. Data scientists need to embrace the big picture to identify problems that often escape notice.
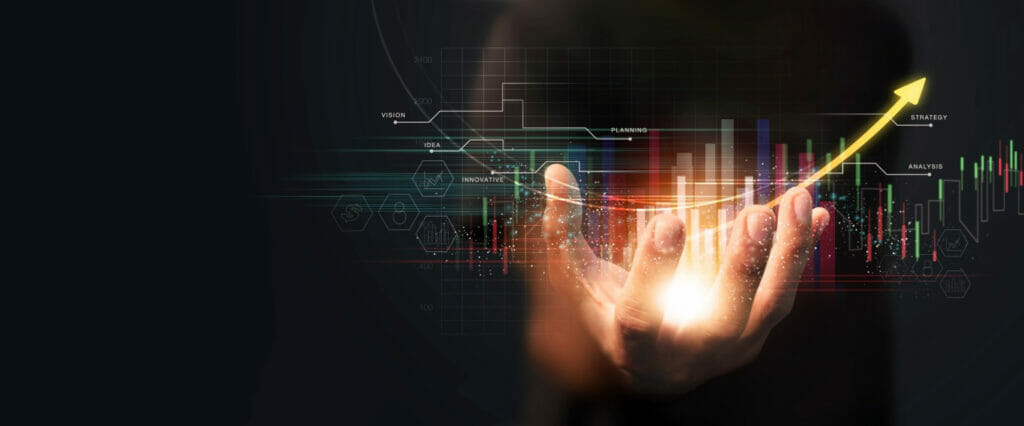
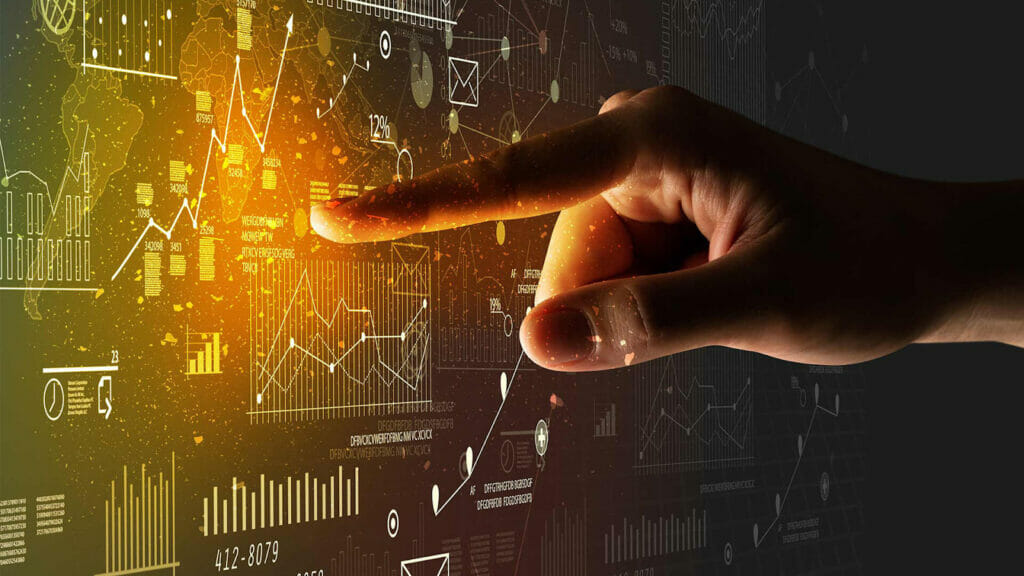
2. Problem Scoping: Gaining Clarity and Specificity
Once you've spotted a problem, the next step is to define its scope clearly. This is crucial because, often, business leaders present challenges in vague terms. They might say something like, "We need to improve customer satisfaction, but we're not sure how." It's the data scientist's job to transform this ambiguity into a well-defined problem.
Effective communication is key here. Data scientists need to ask probing questions like, "What exactly do you mean by 'improve customer satisfaction'?" and "How will we measure success?" By doing this, they can align their analysis with the business goals and select the right data and techniques to solve the problem.
3. Problem Shepherding: Getting Updates, Gathering Feedback
Now, let's talk about problem shepherding. Once you've scoped the problem, it's a mistake to disappear into isolation until you have a solution. Instead, you should empower your team to share preliminary results and gather feedback from business leaders.
Surprises at the end can backfire. If the business team isn't involved throughout the process, they may question the data and methods. It's better to bring them along, making them part of the decision-making process. This collaborative approach ensures that the final results align with the business's expectations.
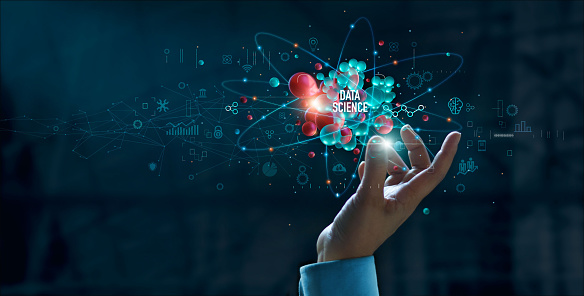
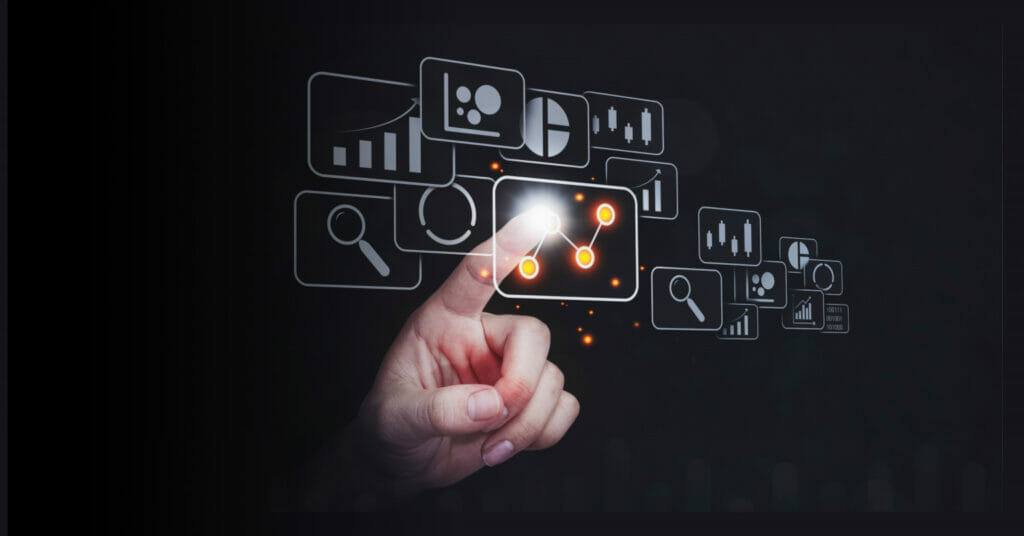
4. Solution Translating: Speaking the Language of the Audience
Finally, after all the hard work, it's time to transition from problem to solution. But remember, it's not just about presenting data; it's about conveying the solution in a language the business team understands.
One powerful tool for this is the two-page data analytics memo. It condenses complex information into a format that's easy to digest. The key here is brevity. By focusing on recommendations and evidence, you can engage business leaders with clear and understandable solutions.
In conclusion, the next generation of data scientists needs to go beyond number crunching. They should be problem spotters, skilled in scoping, effective shepherds, and expert translators. These skills will not only make them invaluable to businesses but also bridge the gap between data and decision-makers.
So, there you have it – four essential skills for the data scientists of the future. What's your take on this, Do you think these skills are spot-on for the evolving field of data science?